Case Studies
Our researchers are using Kelvin2 to tackle a range of complex simulations, data analysis, and modelling in fields such as Neurotechnology, Precision medicine and Metabolomics.
HPC allows them to process large datasets quickly, perform detailed simulations, and solve intricate mathematical problems that would be impractical with standard computing resources.
From neutron star collision to ground breaking cancer research to eco-designing for the future take a look at some of the groundbreaking studies carried out by NI-HPC users!
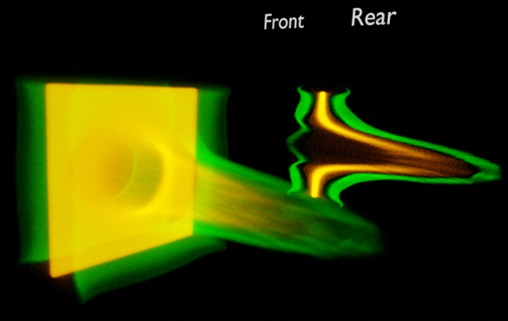
Carbon ion beam to enhance the precision of cancer treatment